Phd Philosophy under Development Deep Neural Network
Intership with The national university of water and Environmental Engineering to develop the web application to diagnosis Eyes diseaes Retina Pathway
End-to-End-integration-Model
this project was collboration with The national university of water and Environmental Engineering to develop the web application to diagnosis Eyes diseaes Retina Pathway , and it was Phd Philosophy under title Development Deep Neural Network for diagnostics Eyes diseaes
- Description Project:
- we worked on End-to-End integration Models that contain tWo models Classification and Segementation each one of it built using different technologies , but important step in any Machine Learning Process is Deployment Model into production , we developed a Web Application using Flask to create two EndPoints REST APIs .
in our Purposal Phd Philosophy under Development Deep Neural Network for diagnostics Eyes diseaes Drive
Part 1 building models:
as follows we built two models classifcation and segmentation and each one asychronized way. </br> Notation : all the models have been used are in Notebooks folder
-
Classification :
- problem description :
at this stage we explored different models architecture in computer vision , and we used Convolution neural network with adding improvement to it
- collecting data : we obtained the data from Kaggle and the team Lab Labeled it manually with expeter and it has Four Classes (DiabeticRetinopathy , Glaucoma , Myopia , Normal)
- Processing : the data was nosiy and we used Per-Processing technique to enhance data such super Resolution (Autoencoder) , Oversampling (DCGAN) , Histogram Equilazation
- built model : we explored different models architecture such RESNET and MobileNEt from Tensoflow Per-Trained models and we funed tune the parameters we reach out wiht out final model to 96.58% AUC and it was based CNN and Smoothing is technic Regulazition to vectorize labels , and all the training process was implemented on Colab Google Platfrom that Provide GPU
- problem description :
at this stage we explored different models architecture in computer vision , and we used Convolution neural network with adding improvement to it
-
Segmentation :
- Problem Description:
after we built the Classifier and test on different sample some problems we faced are :
- similar features between Classes which make the model not able to predict each class good ,
- Map vessel blood of eyes have low pixles range and hard to make model focus on the region of interest. in this stage we used UNET MODEL to Re-Create a new database which only have the Map Vessel Boold ,
- collecing data: the main goal of this model is re-creating segemented image from Funds image to reduce the high value pixle of unsued region and only keep map Vessel Boold of the eye the Dataset
- Built model : we used UNET model architecture since widly used in Biomedical Image segmentation is based on Encoder-Decoder interfaces implemented using Pytorch Framework .
- Problem Description:
after we built the Classifier and test on different sample some problems we faced are :
- Grad-CAM : to make sure the model classification is performed in the right order we applied Grad-CAM activation class map to show the most area the model learn from here the Full Backend Process End-to-End integration
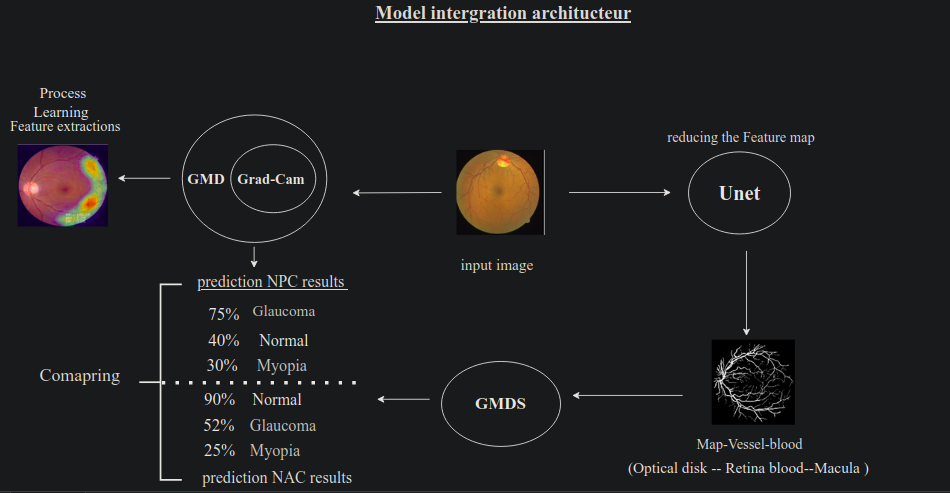
Part 2 deployment MLOps:
next part was deploy the model into production to make easy to use , we developed web application using Flask to create two EndPoints APIS work ascychronously which means goes from segmentation task into classification.the application has two interfaces to upload images
-
First interface : take the image and pass it into the segementer to obtain the map vessel boold and use the classifier to output the probability outcome of diseaes .
-
Second interface :
only has the Classifier whihout need to segmente the image
- the interfaces are the Endpoints RESt APIs :
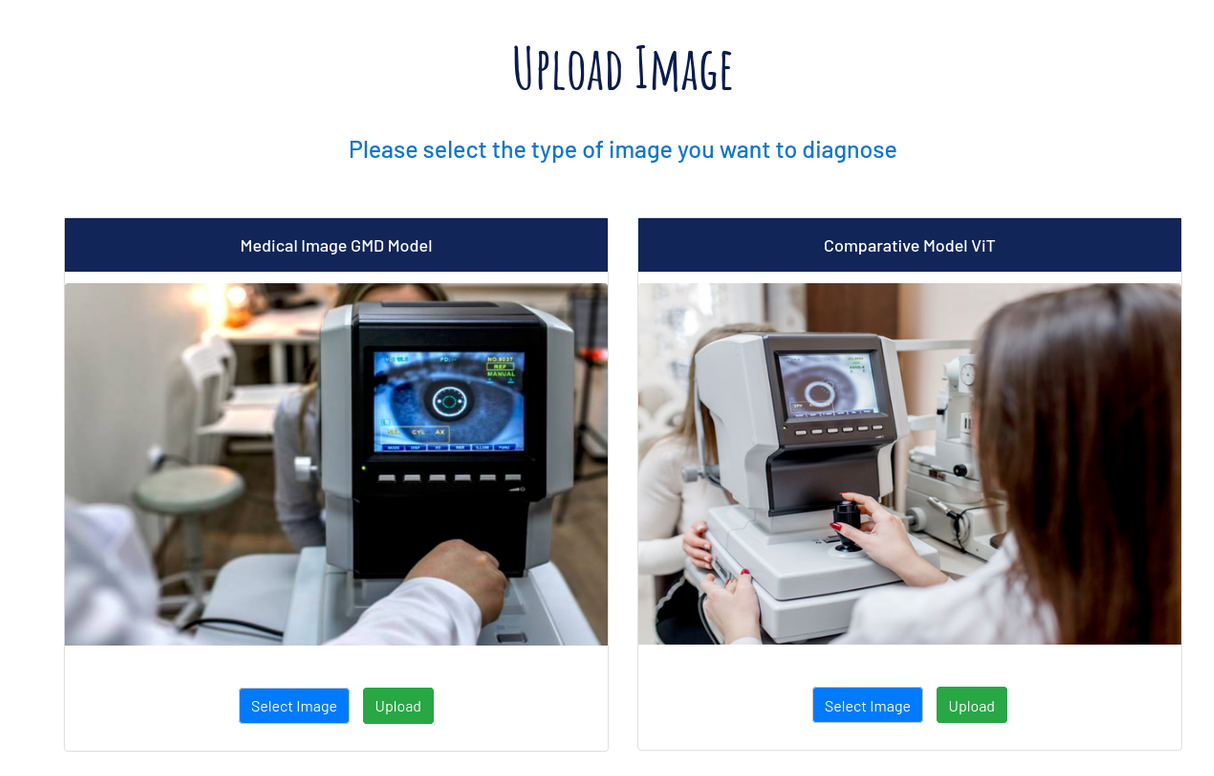
-
Run :
- to run the applicaion , install the reqqirements packages following
pip install -r requirements.txt
- after run the app.py = host the application
python app.py
chose from folder sample/test image to perdict which the first interface will segment the image and save the results in Segement Folder
Following this Project we explored two Reseaches with team from the national university of water and Environmental Engineering to improve performance of models:
References
2021
-
DCGAN for Enhancing Eye Diseases Classification.In CMIS, Fre 2021
-
Medical Image Enhancement Based on Convolutional Denoising Autoencoders and GMD Model.In MoMLeT+ DS, Fre 2021